Welcome aboard another riveting journey into the realms of Databricks! If you’ve been following our previous articles, you’re well on your way to building a solid foundation in Databricks. But fear not if you’ve just joined us — we’re here to catch you up on what you’ve missed. In our earlier stories, we delved into the intricacies of Databricks’ architecture, uncovering its key components that pave the way for creating comprehensive end-to-end machine learning solutions. If you need a refresher, you can find our previous discussion [#1]. Additionally, we took a deep dive into workspace capabilities and workflow definitions, with a particular focus on Jobs [#2].
Now, as promised in the previous article, it’s time to plunge into one of the cornerstone components of Databricks’ workflow — the so-called Delta Live Tables (DLTs). Think of DLTs as a declarative framework that lays the groundwork for building robust, maintainable, and thoroughly testable data processing pipelines for data quality.
Throughout this article, we’ll guide you through the development journey of a DLT, highlighting its core features, and shedding light on their respective benefits and limitations. We’ll also introduce you to a critical aspect of every business solution: data quality. With DLTs’ expectation feature, we’ll set up barriers within our pipelines to ensure that only pristine data flow through the DLT and are stored in the data lake. So, buckle up for our third adventure into the captivating world of Databricks!
1. Syntaxis DLTs
We’ll give you the basis to understand Delta Live Tables syntaxis, so we will have the tools to build our first DLT pipeline. To grasp the Delta Live Tables (DLT) syntax fundamentals and commence constructing our DLT pipeline, we must first import the “dlt” module:
Once imported, it’s crucial to note that both materialized views and streaming tables employ the @table decorator. To load a streaming table, simply apply it to a streaming read operation (table.readStream). Conversely, utilize static read (table.read) for loading a materialized view.
Below you can find the general syntax for declaring DLT materialized views and streaming tables:
For views, Databricks simplifies the process with the @view decorator:
By using a decorator, optional parameters, and a function returning a table, which will be stored in the target schema defined during DLT creation, table declaration becomes straightforward. For a detailed reference of DLT parameters, visit [link]. Additionally, various decorators related to Data Quality facilitate handling “bad” data, as discussed later in this article.
Now that we’ve covered the general DLT syntax, let’s deep into an example illustrating data loading in such pipelines. Typically, the pipeline’s first table retrieves data from the metastore:

Then, within the same pipeline, we can read from the initial table using the declaring function’s name:
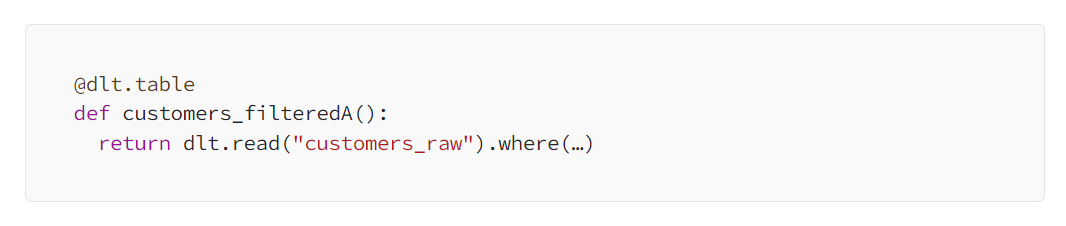
Evidently, working with DLTs proves straightforward. Constructing pipelines to prep data for ML solutions can be achieved succinctly within a single notebook. While we’ve demonstrated the primary operations for loading and storing these tables, numerous other questions remain to be explored concerning these live versions of Delta Tables, such as: What are the DLT types? How do we monitor them? How do we select the target schema? We will try to answer these questions in the following sections.
2. DLT stands for Delta Live Tables
In summary, Delta Live Tables (DLT) in Databricks refers to a comprehensive framework designed for managing and processing data workflows in real-time and batch modes. It can integrate with Delta Lake, enabling efficient data ingestion, transformation, and analysis across various data sources and formats. It has features such as streaming tables, views, and materialized views, enabling users to build scalable data pipelines for diverse use cases, including real-time analytics, machine learning, and operational monitoring. We will describe these features in the following subsections:
Tables and views
In Databricks, Delta Live Tables (DLTs) offer a versatile framework for data processing, featuring three primary methods: streaming tables, views, and materialized views.
- Streaming Tables: Streaming tables are tailored for handling streaming or incremental data processing tasks. They are engineered to efficiently manage growing datasets, ensuring each row is processed only once. This capability is pivotal for ingestion workloads requiring data freshness and low latency, making streaming tables well-suited for real-time data solutions.
- Views: views serve as intermediate object representation within the Delta Live Pipeline. While views can compute or apply caching optimizations, they do not materialize the results. Instead, they optimize data access and processing within the pipeline itself. Databricks advocates for leveraging views to enforce data quality constraints and augment datasets, thus facilitating the efficient execution of multiple downstream queries.
- Materialized Views: Materialized views represent a more concrete form of views, where the result is precomputed according to a specified refresh schedule. These tables are adept at managing any changes in the input data and persisting in the computed output to the metastore or catalog. Materialized views offer a practical solution for scenarios requiring precomputed, frequently accessed data, enhancing query performance and reducing computational overhead.
The image below shows a graphical representation of these three concepts:
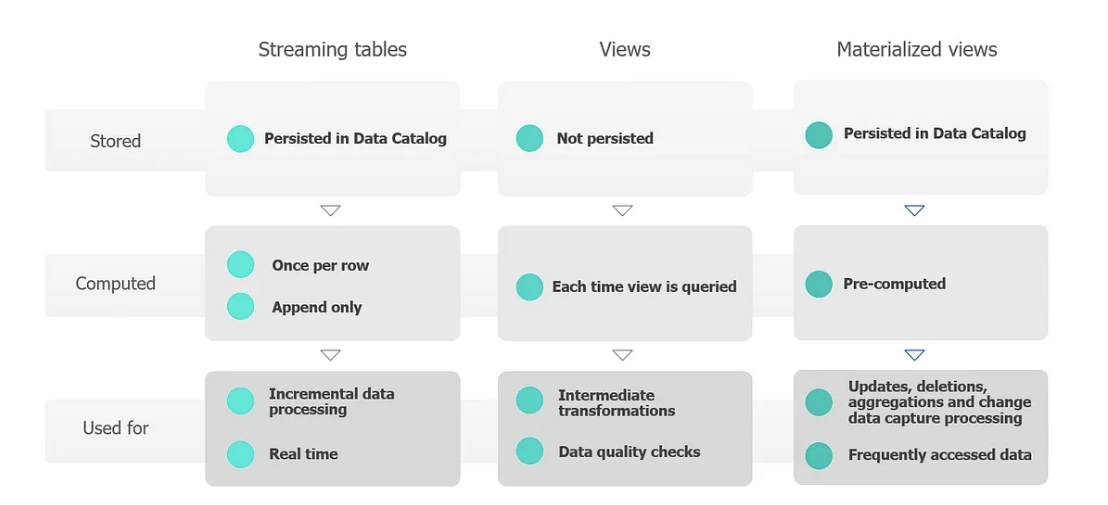
Delta Live Table pipelines
Pipelines comprise materialized views and streaming tables, which are declared in Python or SQL source files. DLT intelligently infers dependencies between these tables, ensuring that updates occur in the correct sequence.
Delta Live Tables pipelines feature two primary categories of settings:
- Dataset Declarations: These configurations define the notebooks or files containing Delta Live Tables syntax for declaring datasets. As demonstrated in the previous section, pipelines are managed through decorators and functions within notebooks.
- Pipeline Infrastructure Settings: These configurations control the pipeline infrastructure, update processing, and table saving within the workspace. This aspect will be further explored in the subsequent section, where we’ll create a DLT pipeline from scratch and configure each necessary step.
While many configurations are optional, some require meticulous attention, particularly for production pipelines. These critical configurations include:
- Target Schema Declaration: Specifying a target schema is essential for publishing data outside the pipeline, especially to the Hive metastore or Unity Catalog.
- Data Access Permissions Configuration: Configuring data access permissions in the execution cluster ensures appropriate access to data sources and target storage locations.
Limitations of DLTs
While Delta Live Tables (DLT) offers powerful capabilities, there are certain limitations to consider:
- Target Schema Constraint: The target schema can only be set for the entire DLT pipeline. This restricts the ability to store output from intermediate steps in the pipeline to different schemas.
- Exclusive Delta Table Usage: All tables created and updated by Delta Live Tables are automatically designated as Delta tables, limiting flexibility in utilizing alternative formats.
- Single Operation Restriction: DLTs can only serve as the target of a single operation within all Delta Live Tables pipelines, potentially constraining complex pipeline configurations.
- Identity Column Limitation: Identity columns cannot be utilized with tables targeted by “APPLY CHANGES INTO” and may undergo re-computation during updates for materialized views. To ensure smooth operations, Databricks recommends restricting the use of identity columns for streaming tables within Delta Live Tables.
- Pipeline Limitation: There is a current limitation of 100 DLT pipelines within a single workspace, which may impact scalability for organizations managing large-scale data workflows.
3. What to expect, data quality?
In any well-defined data project, establishing clear constraints and rules is essential for achieving goals within the boundaries of data quality. Databricks provides a framework for defining this quality using the so-called “expectations”.
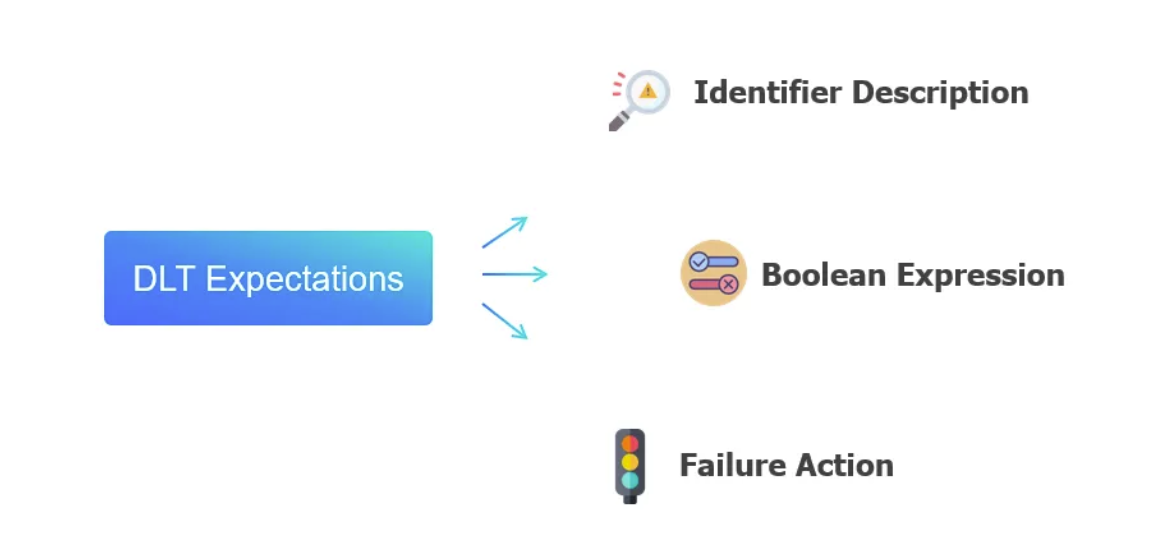
As introduced in Section 1, expectations are optional clauses within your Delta Live Tables (DLT) declaration. These clauses enable engineers to define, manage, and monitor data quality for datasets.
The expectations consist of three primary blocks:
- Identifier Description: This block serves as a unique label, facilitating metric tracking for the specified constraint.
- Boolean Expression: It comprises a logical statement that evaluates to either true or false based on a specified condition.
- Failure Action: This block defines the response to be taken when a record fails to meet the expectation, resulting in the boolean expression returning false.
There are three possible failure actions:

To specify multiple data quality conditions, you can utilize the following options: “expect_all”, “expect_all_or_drop”, and “expect_all_or_fail”:
@dlt.expect_all({"valid_age": "age > 0", "valid_renta_null": "renta IS NOT NULL"})
These simple yet powerful declarations enable you to establish robust data quality checks within an advanced analytics solution. By incorporating these declarations, you can ensure the reliability and integrity of your data throughout the processing pipeline. In the upcoming section, we will apply these principles to construct the pre-processing pipeline introduced in the previous article. This pipeline will not only facilitate the construction of our model but also guarantee the proper processing of data for our machine-learning solution.
4. Example use case
Now equipped with all the information, we will embark on the construction of a Delta Live Table (DLT) pipeline within Databricks. This pipeline will serve to prepare our data for model exploration and pre-processing stages, as described in the past article [#2]. Let’s dive into the technical details starting with the initial steps within the Databricks user interface (UI), followed by the notebook configurations to define the “expectations” and processing methodologies.
To commence, let’s navigate to the Databricks UI and initiate the creation of our DLT pipeline. In the left pane menu, locate and click on “Delta Live Tables”. Once accessed, see the upper-right corner of the page and click on “Create pipeline”.
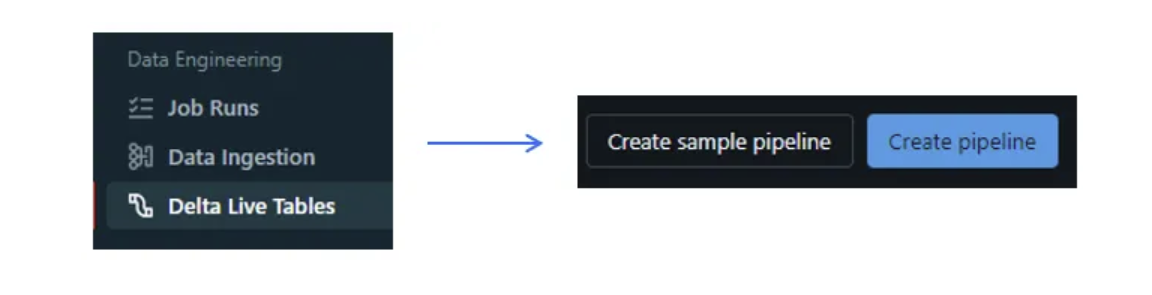
Now that we’ve accessed the DLT pipeline creation user interface, we can configure the primary settings either through the UI or in JSON format. Let’s delve into setting up our first DLT pipeline by focusing on the critical parameters:
- Pipeline Name: For our demonstration, we’ll name it as “product_recommendation_dlt”.
- Source Code: Specify the path to the notebook containing the code of the DLT pipeline.
- Target Schema: It’s essential to select a target schema, noting that only one schema can be chosen. In our case, we’ll opt for the “gold” schema for our solution.
- Min and Max Workers: Determine the range for the number of workers, typically set between 1 and 5 for initial configurations.
Upon completion, click the “Save” button to preserve your settings. Subsequently, you’ll be redirected to the main DLT page, which bears resemblance to the interface of every “Job” page.
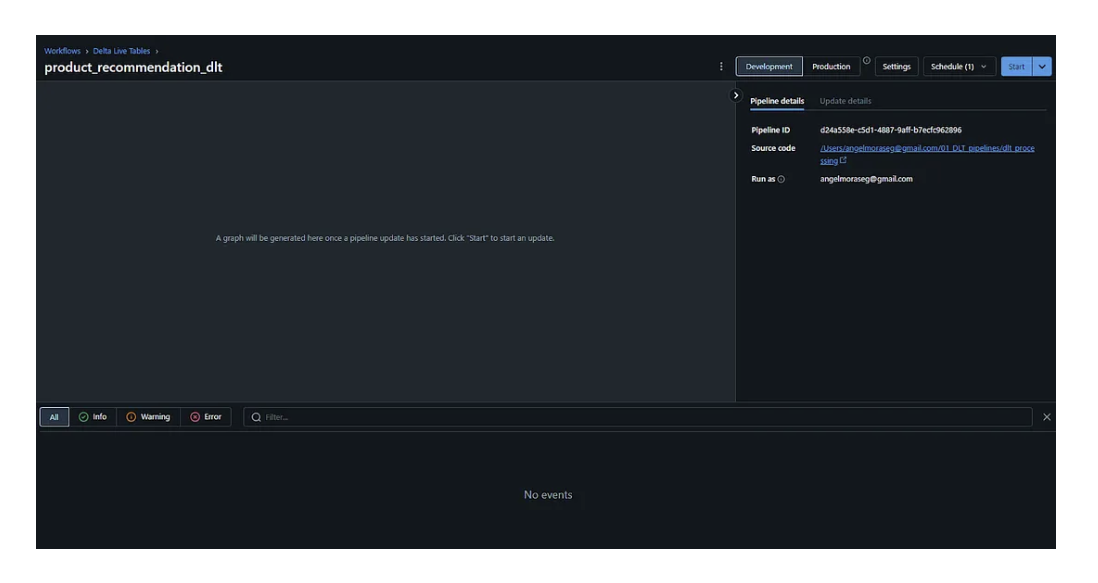
The final step in the UI has been reached. Go to your source code “Notebook” and let’s start coding!
We will manage our DLT using three main cells; one per layer: bronze, silver, and gold. In the first cell, we will load the data and apply a general check on nulls, empty strings, or wrong values. To apply this check over all possible columns we will create an auxiliary function like this:
columns_list = spark.read.table("bronze.raw_products_santander").columns
expectations = {}
for i in columns_list:
expectations["valid_" + i] = f"{i} IS NOT NULL OR {i} <> \"\" OR {i} <> \"NA\""
The result looks like this:
Then we will create the first step of our DLT using “expectations” as the check to be done. In this first step, we assume some wrong values will reach the raw state of data. Therefore, we will just notify you in case the data quality is not being met.
In this first step, we won’t do anything as we would like our raw data to be kept as pure as it comes.

In the second step, we will apply the same data quality check, but now we will apply some rules to “fix” these wrong data.
@dlt.expect_all(expectations)
@dlt.create_table(
comment="The products separated into facts and master. With DQ applied. No nulls from this point",
table_properties={
"quality": "silver",
"pipelines.autoOptaimize.managed": "true",
"skipChangeCommits": "true",
},
)
def silver_products_recommendation():
df = (
dlt.read_stream("bronze_products_recommendation")
.withColumn(
"age",
when(
(col("age") == "NA") | (col("age") == "") | (col("age").isNull()),
mean_age,
)
.when(col("age") < min_age, min_age)
.when(col("age") > max_age, max_age)
.otherwise(col("age")),
)
.withColumn(
"renta",
when(
(col("renta") == "NA") | (col("renta") == "") | (col("renta").isNull()),
missing_renta,
)
.when(col("renta") < min_renta, min_renta)
.when(col("renta") > max_renta, max_renta)
.otherwise(col("renta")),
)
.withColumn(
"antiguedad",
when(
(col("antiguedad") == "NA")
| (col("antiguedad") == "")
| (col("antiguedad").isNull()),
missing_antiguedad,
)
.when(col("antiguedad") < min_antiguedad, min_antiguedad)
.when(col("antiguedad") > max_antiguedad, max_antiguedad)
.otherwise(col("antiguedad")),
)
)
for cat_col in cat_cols:
df = df.withColumn(
cat_col,
when(
(col(cat_col) == "NA") | (col(cat_col) == "") | (col(cat_col).isNull()),
-99,
).otherwise(col(cat_col)),
)
for product in product_list:
df = df.withColumn(
product,
when(
(col(product) == "NA") | (col(product) == "") | (col(product).isNull()),
0,
)
.otherwise(col(product))
.cast("int"),
)
return df
Finally, the same check will be done, but this time we would like to add some new features to the gold table. These features will enrich the future ML solution.
@dlt.expect_all(expectations)
@dlt.create_table(
comment="The products with added features. getRent, getAge, getSeniority",
table_properties={
"quality": "gold",
"pipelines.autoOptimize.managed": "true",
},
)
def gold_products_recommendation():
df = (
dlt.read_stream("silver_products_recommendation")
.withColumn("age_norm", round((col("age") - min_age) / range_age, 4))
.withColumn("renta_norm", round((col("renta") - min_renta) / range_renta, 6))
.withColumn(
"antiguedad_norm",
round((col("antiguedad") - min_antiguedad) / range_antiguedad, 4),
)
)
return df
We’ve reached the end of our DLT pipeline development. Let’s come back again to the DLT page, where we will be able to run it. You will see the DLT process during its execution:
Once it is finished, let’s look into the Data Quality checks made to validate that our data processing is filling up the null values properly.
Starting with the bronze step, we detect how multiple columns are raising the “warn” of wrong data entering the pipeline.
However, if we jump to the silver or gold layer, our data processing eliminates most of these issues:
For this last field, we will consider our data quality checks to apply the necessary pre-processing to our model. Since the information this field is giving us is quite insignificant, we will remove the column from later on layers.
Finally, you will find the results in the metastore, where our three layers are stored under the gold schema (remember one of the DLT limitations was to not to be able to store each layer step into different schemas).
Conclusions
The integration of Delta Live Tables for data quality rules in Databricks heralds a paradigm shift in ensuring data reliability and integrity. With Delta Live Tables, organizations can define and implement data quality rules using a straightforward and intuitive syntax within their Databricks environment. Also, they can monitor its execution using jobs integrated with the rest of Databricks’ workflows. Thus, DLT’s syntax allows data teams to express complex data quality requirements in a concise and easily understandable manner.
Whether it’s validating data types, enforcing referential integrity, or detecting anomalies, the expressive nature of the syntax enables teams to articulate their requirements with simplicity and clarity. Moreover, the integration of Delta Live Tables with Delta Lake architecture ensures that these data quality rules are enforced consistently and efficiently across diverse data pipelines. This approach not only mitigates the risk of data errors but also fosters a culture of data-driven decision-making based on accurate and reliable insights.
References
For this article, we have used the following references:
- Databricks. (2023, October 12). Delta Live Tables Guide. https://docs.databricks.com/en/delta-live-tables/index.html
- Microsoft Azure. (n.d.). Delta Live Tables — Publish. https://learn.microsoft.com/en-gb/azure/databricks/delta-live-tables/publish
- Databricks. (n.d.). Python Delta Live Tables Properties. https://docs.databricks.com/en/delta-live-tables/python-ref.html#python-delta-live-tables-properties
- Databricks. (n.d.). Delta Live Tables Python Reference. https://docs.databricks.com/en/delta-live-tables/python-ref.html
- Databricks. (n.d.). Delta Live Tables Expectations. https://docs.databricks.com/en/delta-live-tables/expectations.html
- Stack Overflow. (2021, June 5). Move managed DLT tables from one schema to another schema in Databricks. https://stackoverflow.com/a/76876914
Autores
- Gonzalo Zabala
Senior Consultant SDG Group
- Ángel Mora
Specialist Lead SDG Group